JNTU-K B.TECH R19 4-2 Syllabus For Deep learning PDF 2022
February 1, 2022 2022-02-01 17:28JNTU-K B.TECH R19 4-2 Syllabus For Deep learning PDF 2022
JNTU-K B.TECH R19 4-2 Syllabus For Deep learning PDF 2022
Get Complete Lecture Notes for Deep learning on Cynohub APP
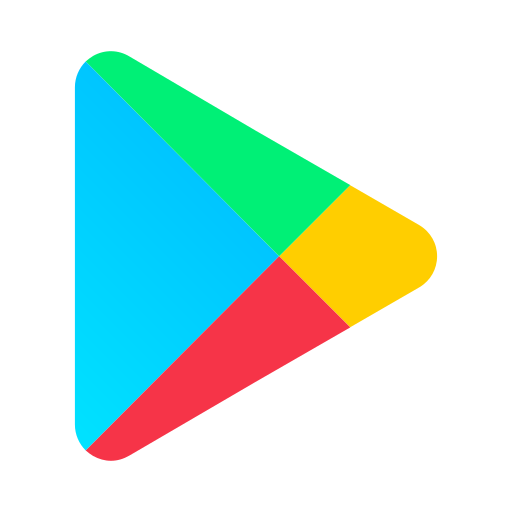
Download the APP Now! ( Click Here )
You will be able to find information about Deep learning along with its Course Objectives and Course outcomes and also a list of textbook and reference books in this blog.You will get to learn a lot of new stuff and resolve a lot of questions you may have regarding Deep learning after reading this blog. Deep learning has 5 units altogether and you will be able to find notes for every unit on the CynoHub app. Deep learning can be learnt easily as long as you have a well planned study schedule and practice all the previous question papers, which are also available on the CynoHub app.
All of the Topic and subtopics related to Deep learning are mentioned below in detail. If you are having a hard time understanding Deep learning or any other Engineering Subject of any semester or year then please watch the video lectures on the official CynoHub app as it has detailed explanations of each and every topic making your engineering experience easy and fun.
Deep learning Unit One
Linear Algebra
Linear Algebra: Scalars, Vectors, Matrices and Tensors, Matrix operations, types of matrices, Norms, Eigen decomposition, Singular Value Decomposition, Principal Components Analysis.
Probability and Information Theory: Random Variables, Probability Distributions, Marginal Probability, Conditional Probability, Expectation, Variance and Covariance, Bayes’ Rule, Information Theory. Numerical Computation: Overflow and Underflow, Gradient-Based Optimization, Constrained Optimization, Linear Least Squares.
Deep learning Unit Two
Machine Learning
Machine Learning: Basics and Underfitting, Hyper parameters and Validation Sets, Estimators, Bias and Variance, Maximum Likelihood, Bayesian Statistics, Supervised and Unsupervised
Learning, Stochastic Gradient Descent, Challenges Motivating Deep Learning. Deep Feedforward Networks: Learning XOR, Gradient-Based Learning, Hidden Units, Architecture Design, Back-Propagation and other Differentiation Algorithms.
Get Complete Lecture Notes for Deep learning on Cynohub APP
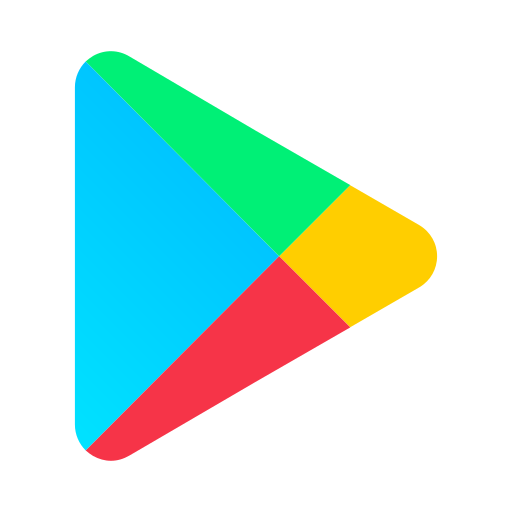
Download the APP Now! ( Click Here )
Deep learning Unit Three
Regularization for Deep Learning
Regularization for Deep Learning: Parameter Norm Penalties, Norm Penalties as Constrained
Optimization, Regularization and Under-Constrained Problems, Dataset Augmentation, Noise Robustness, Semi-Supervised Learning, Multi-Task Learning, Early Stopping, Parameter Tying and Parameter Sharing, Sparse Representations, Bagging and Other Ensemble Methods, Dropout, Adversarial Training, Tangent Distance, Tangent Prop and Manifold Tangent
Classifier. Optimization for Training Deep Models: Pure Optimization, Challenges in Neural
Network Optimization, Basic Algorithms, Parameter Initialization Strategies, Algorithms with Adaptive Learning Rates, Approximate Second-Order Methods, Optimization Strategies and Meta-Algorithms.
Deep learning Unit Four
Convolutional Networks
Convolutional Networks: The Convolution Operation, Pooling, Convolution, Basic Convolution Functions, Structured Outputs, Data Types, Efficient Convolution Algorithms, Random or Unsupervised Features, Basis for Convolutional Networks.
Deep learning Unit Five
Sequence Modeling
Sequence Modeling: Recurrent and Recursive Nets: Unfolding Computational Graphs, Recurrent
Neural Networks, Bidirectional RNNs, Encoder-Decoder Sequence-to-Sequence Architectures, Deep Recurrent Networks, Recursive Neural Networks, Echo State Networks, LSTM, Gated RNNs, Optimization for Long-Term Dependencies, Auto encoders, Deep Generative Models.
Deep learning Course Objectives
Demonstrate the major technology trends driving Deep Learning
Build, train and apply fully connected deep neural networks
Implement efficient (vectorized) neural networks
Analyze the key parameters and hyper parameters in a neural network’s architecture
Deep learning Course Outcomes
Demonstrate the mathematical foundation of neural network
Describe the machine learning basics
Differentiate architecture of deep neural network
Build a convolutional neural network
Build and train RNN and LSTMs
Deep learning Text Books
1) Ian Goodfellow, Yoshua Bengio, Aaron Courville, “Deep Learning”, MIT Press,2016.
2) Josh Patterson and Adam Gibson, “Deep learning: A practitioner’s approach”, O’Reilly Media, First Edition, 2017.
Deep learning Reference Books
1) Fundamentals of Deep Learning, Designing next-generation machine intelligence algorithms, Nikhil Buduma, O’Reilly, Shroff Publishers, 2019.
2) Deep learning Cook Book, Practical recipes to get started Quickly, Douwe Osinga, O’Reilly, Shroff Publishers, 2019.
Scoring Marks in Deep learning
Scoring a really good grade in Deep learning is a difficult task indeed and CynoHub is here to help!. Please watch the video below and find out how to get 1st rank in your B.tech examinations . This video will also inform students on how to score high grades in Deep learning. There are a lot of reasons for getting a bad score in your Deep learning exam and this video will help you rectify your mistakes and help you improve your grades.
Information about JNTU-K B.Tech R19 Deep learning was provided in detail in this article. To know more about the syllabus of other Engineering Subjects of JNTUH check out the official CynoHub application. Click below to download the CynoHub application.
Get Complete Lecture Notes for Deep learning on Cynohub APP
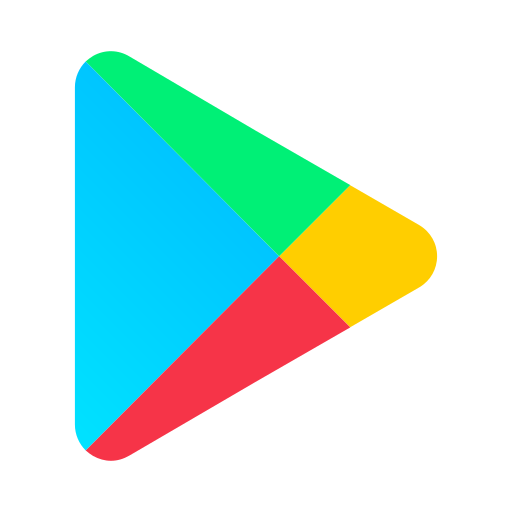