JNTU-K B.TECH R19 4-1 Syllabus For Neural networks and fuzzy logic PDF 2022
February 1, 2022 2022-02-01 20:48JNTU-K B.TECH R19 4-1 Syllabus For Neural networks and fuzzy logic PDF 2022
JNTU-K B.TECH R19 4-1 Syllabus For Neural networks and fuzzy logic PDF 2022
Get Complete Lecture Notes for Neural networks and fuzzy logic on Cynohub APP
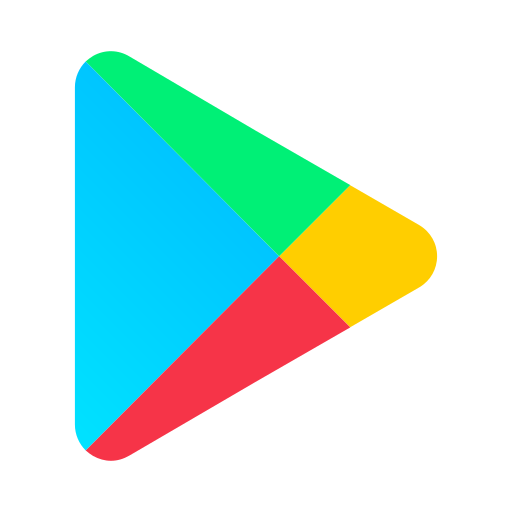
Download the APP Now! ( Click Here )
You will be able to find information about Neural networks and fuzzy logic along with its Course Objectives and Course outcomes and also a list of textbook and reference books in this blog.You will get to learn a lot of new stuff and resolve a lot of questions you may have regarding Neural networks and fuzzy logic after reading this blog. Neural networks and fuzzy logic has 5 units altogether and you will be able to find notes for every unit on the CynoHub app. Neural networks and fuzzy logic can be learnt easily as long as you have a well planned study schedule and practice all the previous question papers, which are also available on the CynoHub app.
All of the Topic and subtopics related to Neural networks and fuzzy logic are mentioned below in detail. If you are having a hard time understanding Neural networks and fuzzy logic or any other Engineering Subject of any semester or year then please watch the video lectures on the official CynoHub app as it has detailed explanations of each and every topic making your engineering experience easy and fun.
Neural networks and fuzzy logic Unit One
Introduction
Artificial Neural Networks (ANN) – Humans and computers – Biological neural networks – ANN Terminology – Models of Artificial neuron – activation functions – typical architectures – biases and thresholds – learning strategy(supervised, unsupervised and reinforced) – Neural networks learning rules. Single layer feed forward neural networks: concept of pattern and its types, perceptron training and classification using Discrete and Continuous perceptron algorithms– linear separability- XOR function.
Neural networks and fuzzy logic Unit Two
ANN Paradigms
Multi-layer feed forward networks –Generalized delta rule– Back Propagation algorithm – Radial Basis Function (RBF) network. Kohonen’s self organizing feature maps (KSOFM), Learning Vector Quantization (LVQ)– Functional Link Networks (FLN) – Bidirectional Associative Memory (BAM) – Hopfield Neural Network.
Get Complete Lecture Notes for Neural networks and fuzzy logic on Cynohub APP
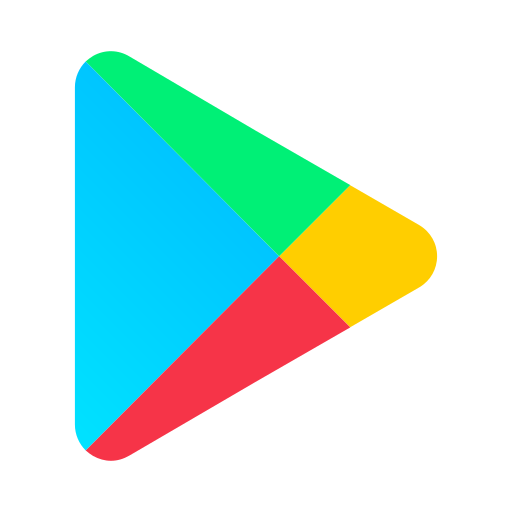
Download the APP Now! ( Click Here )
Neural networks and fuzzy logic Unit Three
Classical and Fuzzy Sets
Introduction to classical sets- properties, Operations and relations; Fuzzy sets, Membership, Operations, Properties, Fuzzy relations, Cardinalities, Membership functions.
Neural networks and fuzzy logic Unit Four
Fuzzy Logic Modules
Fuzzification, Membership value assignment, development of rule base and decision making system, Defuzzification to crisp sets, Defuzzification methods.
Neural networks and fuzzy logic Unit Five
Applications
Neural network applications: Load flow studies, load forecasting, reactive power control. Fuzzy logic applications: Economic load dispatch, speed control of DC motors, single area and two area load frequency control.
Neural networks and fuzzy logic Course Objectives
To understand artificial neuron models & learning methods of ANN.
To utilize different algorithms of ANN.
To distinguish between classical and fuzzy sets.
To understand different modules of fuzzy controller.
To understand applications of neural networks and fuzzy logic.
Neural networks and fuzzy logic Course Outcomes
After the completion of the course the student should be able to:
know different models of artificial neuron & Use learning methods of ANN.
use different paradigms of ANN.
classify between classical and fuzzy sets.
use different modules of Fuzzy logic controller.
apply Neural Networks and fuzzy logic for real-time applications.
Neural networks and fuzzy logic Text Books
1. Introduction to Artificial Neural Systems – Jacek M. Zuarda, Jaico Publishing House, 1997.
2. Neural Networks, Fuzzy logic, Genetic algorithms: synthesis and applications by RajasekharanandPai – PHI Publication.
Neural networks and fuzzy logic Reference Books
1. Artificial Neural Network – B.Yegnanarayana, PHI, 2012.
2. Fuzzy logic with Fuzzy Applications – T.J Ross – Mc Graw Hill Inc, 1997.
3. Introduction to Neural Networks using MATLAB 6.0 – S N Sivanandam,SSumathi,S N Deepa
TMGH
4. Introduction to Fuzzy Logic using MATLAB – S N Sivanandam,SSumathi,S N Deepa Springer, 2007.
Scoring Marks in Neural networks and fuzzy logic
Scoring a really good grade in Neural networks and fuzzy logic is a difficult task indeed and CynoHub is here to help!. Please watch the video below and find out how to get 1st rank in your B.tech examinations . This video will also inform students on how to score high grades in Neural networks and fuzzy logic. There are a lot of reasons for getting a bad score in your Neural networks and fuzzy logic exam and this video will help you rectify your mistakes and help you improve your grades.
Information about JNTU-K B.Tech R19 Neural networks and fuzzy logic was provided in detail in this article. To know more about the syllabus of other Engineering Subjects of JNTUH check out the official CynoHub application. Click below to download the CynoHub application.
Get Complete Lecture Notes for Neural networks and fuzzy logic on Cynohub APP
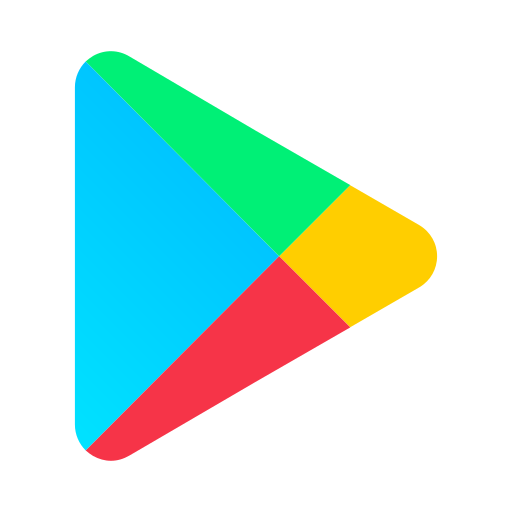