JNTU-K B.TECH R19 4-1 Syllabus For Data science PDF 2022
February 1, 2022 2022-02-01 16:44JNTU-K B.TECH R19 4-1 Syllabus For Data science PDF 2022
JNTU-K B.TECH R19 4-1 Syllabus For Data science PDF 2022
Get Complete Lecture Notes for Data science on Cynohub APP
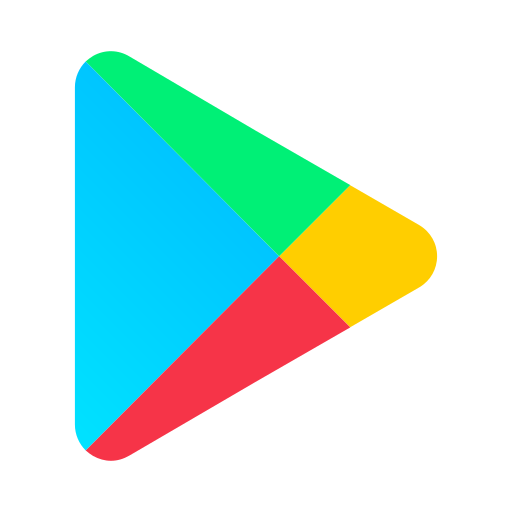
Download the APP Now! ( Click Here )
You will be able to find information about Data science along with its Course Objectives and Course outcomes and also a list of textbook and reference books in this blog.You will get to learn a lot of new stuff and resolve a lot of questions you may have regarding Data science after reading this blog. Data science has 5 units altogether and you will be able to find notes for every unit on the CynoHub app. Data science can be learnt easily as long as you have a well planned study schedule and practice all the previous question papers, which are also available on the CynoHub app.
All of the Topic and subtopics related to Data science are mentioned below in detail. If you are having a hard time understanding Data science or any other Engineering Subject of any semester or year then please watch the video lectures on the official CynoHub app as it has detailed explanations of each and every topic making your engineering experience easy and fun.
Data science Unit One
Introduction
Introduction, The Ascendance of Data, Motivating Hypothetical: Data Sciencester, Finding Key Connectors, The Zen of Python, Getting Python, Virtual Environments, Whitespace Formatting,
Modules, Functions, Strings, Exceptions, Lists, Tuples, Dictionaries defaultdict, Counters, Sets,
Control Flow, Truthiness, Sorting, List Comprehensions, Automated Testing and assert, Object-Oriented Programming, Iterables and Generators, Randomness, Regular Expressions, Functional Programming, zip and Argument Unpacking, args and kwargs, Type Annotations, How to Write Type Annotations.
Data science Unit Two
Visualizing Data
Visualizing Data: matplotlib, Bar Charts, Line Charts, Scatterplots. Linear Algebra: Vectors, Matrices, Statistics: Describing a Single Set of Data, Correlation, Simpson’s Paradox, Some Other Correlational Caveats, Correlation and Causation.
Gradient Descent: The Idea Behind Gradient Descent, Estimating the Gradient, Using the Gradient, Choosing the Right Step Size, Using Gradient Descent to Fit Models, Minibatch and Stochastic Gradient Descent.
Get Complete Lecture Notes for Data science on Cynohub APP
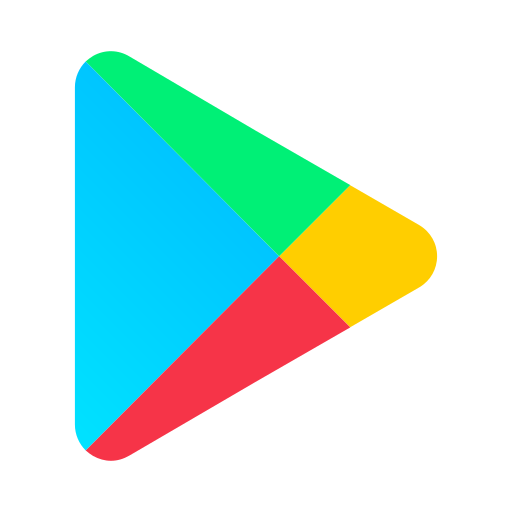
Download the APP Now! ( Click Here )
Data science Unit Three
Getting Data
Getting Data: stdin and stdout, Reading Files, Scraping the Web, Using APIs,
Working with Data: Exploring Your DataUsing NamedTuples, Dataclasses, Cleaning and Munging, Manipulating Data, Rescaling, Dimensionality Reduction.
Probability: Dependence and Independence, Conditional Probability, Bayes’s Theorem, Random
Variables, Continuous Distributions, The Normal Distribution, The Central Limit Theorem
Data science Unit Four
Machine Learning
Machine Learning: Modeling, Overfitting and Underfitting, Correctness, The Bias-Variance
Tradeoff, Feature Extraction and Selection, k-Nearest Neighbors, Naive Bayes, Simple Linear Regression, Multiple Regression, Digression, Logistic Regression
Data science Unit Five
Clustering
Clustering: The Idea, The Model, Choosing k, Bottom-Up Hierarchical Clustering.
Recommender Systems: Manual Curation, Recommending What’s Popular, User-Based
Collaborative Filtering, Item-Based Collaborative Filtering, Matrix Factorization
Data Ethics, Building Bad Data Products, Trading Off Accuracy and Fairness, Collaboration,
Interpretability, Recommendations, Biased Data, Data Protection IPython, Mathematics, NumPy, pandas, scikit-learn, Visualization, R
Data science Course Objectives
From the course the student will learn
Provide you with the knowledge and expertise to become a proficient data scientist
Demonstrate an understanding of statistics and machine learning concepts that are vital for data science
Learn to statistically analyze a dataset
Explain the significance of exploratory data analysis (EDA) in data science
Critically evaluate data visualizations based on their design and use for communicating stories from data
Data science Course Outcomes
At the end of the course, student will be able to
Describe what Data Science is and the skill sets needed to be a data scientist
Illustrate in basic terms what Statistical Inference means. Identify probability distributions
commonly used as foundations for statistical modelling, Fit a model to data
Use R to carry out basic statistical modeling and analysis
Apply basic tools (plots, graphs, summary statistics) to carry out EDA
Describe the Data Science Process and how its components interact
Use APIs and other tools to scrap the Web and collect data
Apply EDA and the Data Science process in a case study
Data science Text Books
1) Joel Grus, “Data Science From Scratch”, OReilly. 2) Allen B.Downey, “Think Stats”, OReilly.
Data science Reference Books
1) Doing Data Science: Straight Talk From The Frontline, 1st Edition, Cathy O’Neil and Rachel Schutt, O’Reilly, 2013
2) Mining of Massive Datasets, 2nd Edition, Jure Leskovek, Anand Rajaraman and Jeffrey Ullman, v2.1, Cambridge University Press, 2014
3) “The Art of Data Science”, 1st Edition, Roger D. Peng and Elizabeth matsui, Lean Publications, 2015
4) “Algorithms for Data Science”, 1st Edition, Steele, Brian, Chandler, John, Reddy,
Swarna, springers Publications, 2016
Scoring Marks in Data science
Scoring a really good grade in Data science is a difficult task indeed and CynoHub is here to help!. Please watch the video below and find out how to get 1st rank in your B.tech examinations . This video will also inform students on how to score high grades in Data science. There are a lot of reasons for getting a bad score in your Data science exam and this video will help you rectify your mistakes and help you improve your grades.
Information about JNTU-K B.Tech R19 Data science was provided in detail in this article. To know more about the syllabus of other Engineering Subjects of JNTUH check out the official CynoHub application. Click below to download the CynoHub application.
Get Complete Lecture Notes for Data science on Cynohub APP
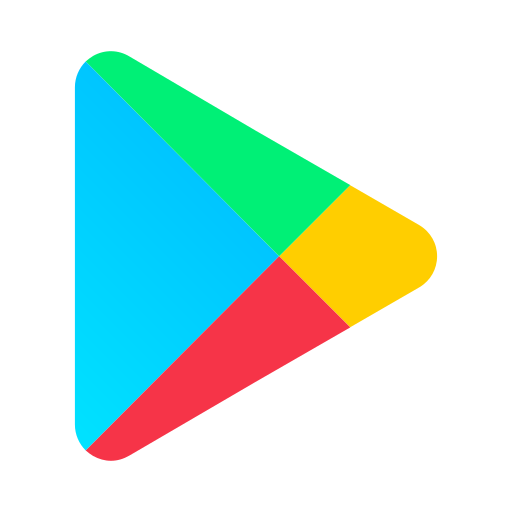